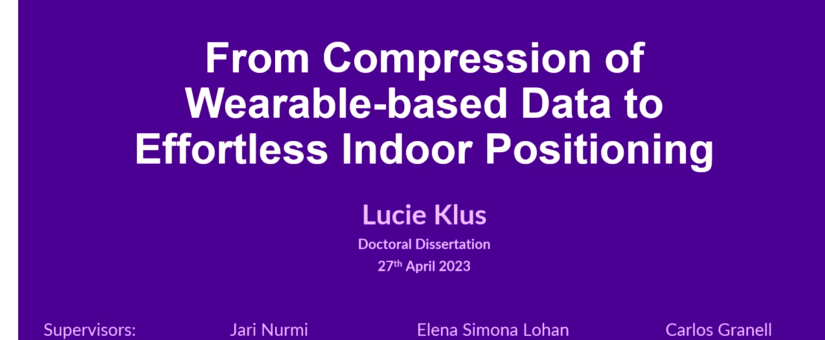
Lucie defended her thesis on wearable data compression techniques
On April 27th, Lucie Klus defended her PhD thesis entitled ‘From Compression of Wearable-based Data to Effortless Indoor Positioning‘ under the supervision of Jari Nurmi and Simona form Tampere University and Carlos Granell. This thesis is part of the cohort of ESR from the European Joint Doctorate Marie Sklodowska-Curie in A Network for Dynamic Wearable Applications with Privacy Constraints (A-WEAR).
Lucie defended her thesis in Tampere, which follows a different assessment from what we do here in Spain. Before the defense, two examiners Prof. Fernando Javier Álvarez Franco (Universidad de Extremadura, Spain) and Dr. Christos Laoudias (University of Cyprus, Cyprus) provided written feedback to the thesis. During the defense today, once Lucie briefly presented her work, only two opponents, Dr. Tobias Feigl (FAU Erlangen-Nürnberg, Germany ) and Dr. Christos Laoudias, were present in the board. All three engaged in a interesting, vivid and scientifically sound discussion on several observations related to the Lucie’s work.
Lucie’s work provides a number of contributions in the field of compression of wearable-based data, mainly in areas of lossy compression techniques designated for the time series sensor-based data and positioning. In particular, two novel time-series compression techniques were proposed, namely Direct Lightweight Temporal Compression (DLTC) and Altered Symbolic Aggregate Approximation (ASAX), which were specifically designed to address relevant challenges of modern wearable systems.
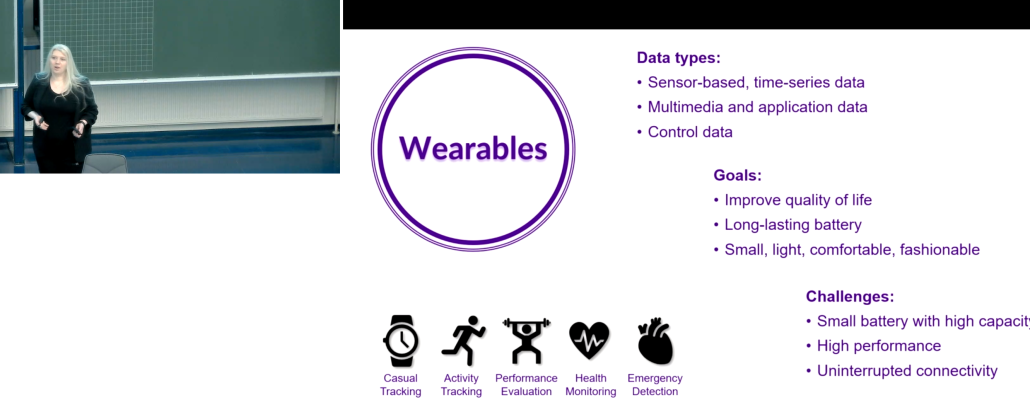
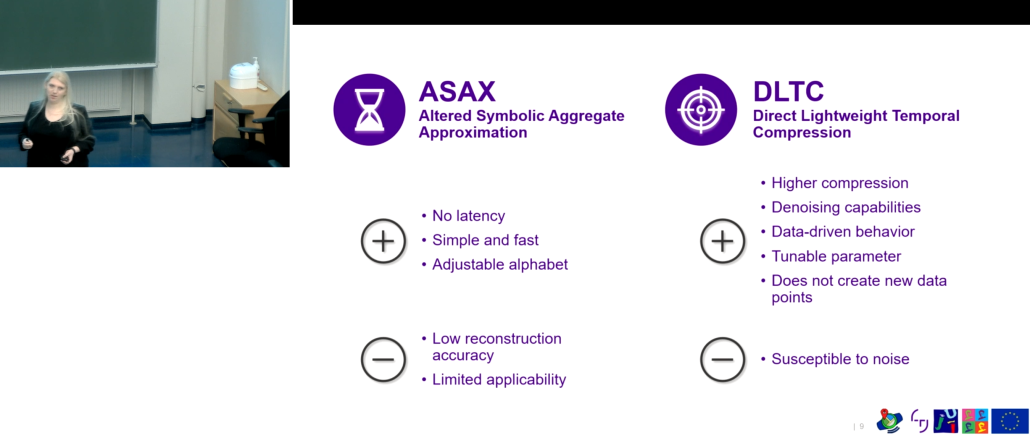
Performing indoor positioning quickly and efficiently on all connected devices, including wearables, becomes crucial in industrial applications, eHealth, or security. Reducing the computational and storage demands for indoor positioning applications was the second challenge addressed. Novel lossy data compression and dimensionality-reduction techniques were also proposed, such as Element-Wise cOmpression using K-means (EWOK), a bitlevel compression based on element-wise k-means clustering, and radio Map compression Employing Signal Statistics (MESS), a sample-wise compression that extracts signal statistics based on their locations.
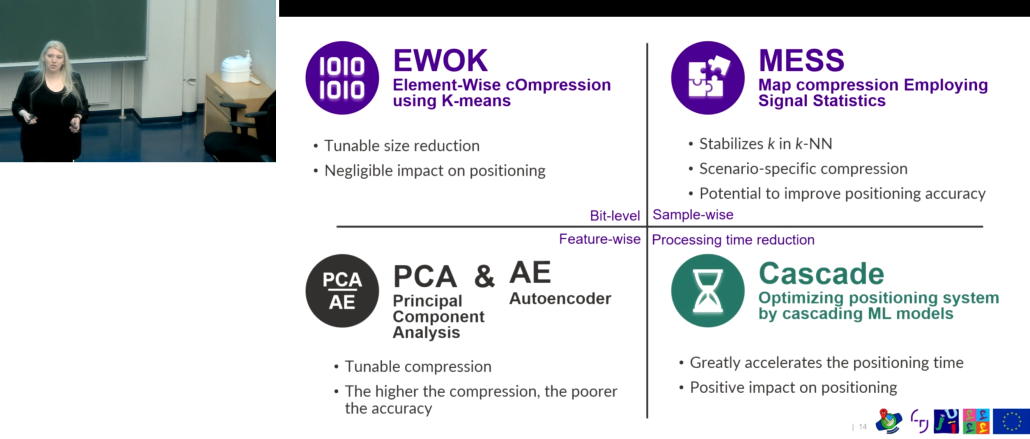
Overall, Lucie’s work provides a relevant number of novel and efficient solutions well beyond the state-of-the-Art in the field of compression of wearable-based data. Enjoy the party. You deserve it!
- Posted by geoadmin
- On 27 April, 2023
- 0 Comments
0 Comments