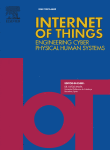
Two @GEOTECUJI papers out in Internet of Thing journal!
Preliminary results of the recently started national research project “Platform for Artificial Intelligence usage on the Internet of Things through the sharing of local micro models between device communities”, in short ColoTIA, with grant reference PID2022-141813OB-I00, have been published in a couple of journal articles in the Elsevier Internet of Things journal.
The first article is “Anomaly detection based on Artificial Intelligence of Things: A Systematic Literature Mapping“, co-authored by Sergio Trilles, Sahibzada Saadoon Hammad and Ditsuhi Iskandaryan. This literature review explores the intersection of Artificial Intelligence of Things (AIoT) and Edge Computing (EC), focusing on the application of advanced Machine Learning (ML) algorithms for anomaly detection in IoT devices. In particular, the study analyses Machine/Deep Learning models on MicroController Units (MCUs). The research identifies 18 key papers published between 2021 and 2023, offering a comprehensive overview of anomaly detection techniques using TinyML and MCUs.

The societal implications of this study lie in the potential benefits and challenges associated with implementing anomaly detection on IoT devices. The integration of accurate data from the initial stage ensures reliable results, contributing to the overall efficiency and effectiveness of AIoT systems. The article delves into various aspects, including the exploration of techniques for anomaly detection, validation metrics used in AIoT, analysis of data for model estimation, and the application of ML in EC using both hardware and software.
The second article published in the same volume of Internet of Thing is “Machine learning-based prediction model for battery levels in IoT devices using meteorological variables“, co-authored by Juan Emilio Zurita Macias and Sergio Trilles. This study addresses a crucial aspect of sustainable technology – the efficient energy management of IoT devices powered by solar harvesting systems. The focus is on preventing battery depletion during periods of low solar incidence. Using a structured methodology called CRISP-DM, the research study introduces machine learning (ML) models that leverage meteorological data to predict battery charge levels in solar-powered IoT devices. This predictive capability allows for proactive adjustments to the devices’ data sampling frequencies, ensuring optimal energy utilization.

The impact on society is significant, as this approach has the potential to enhance the sustainability of IoT devices. By accurately predicting battery levels through the integration of meteorological variables, the models contribute to effective energy autonomy. The study’s empirical results showcase the high accuracy of the ML models, reaching up to 94.09% in specific test cases. This validates the methodology’s ability to substantially improve the energy efficiency of IoT devices, paving the way for more reliable and sustainable technology in our everyday lives.
- Posted by geoadmin
- On 18 February, 2024
- 0 Comments
0 Comments